MIT Engineers Release Massive Open-Source Dataset to Accelerate Eco-Friendly Vehicle Development
MIT engineers have developed the largest open-source dataset of car designs, including their aerodynamics, to accelerate the development of eco-friendly cars and electric vehicles. The dataset, called DrivAerNet++, contains over 8,000 car designs generated based on the most common types of cars in the world today. Each design is represented in 3D form and includes information on the car’s aerodynamics, simulated using computational fluid dynamics.
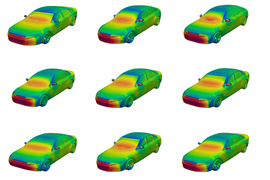
The dataset is designed to be used with generative AI tools, which can quickly process large amounts of data and generate novel designs. By making this dataset publicly available, the researchers aim to promote efficient design processes, cut R&D costs, and drive advancements toward a more sustainable automotive future.
Filling the Data Gap in Car Design
Car design is an iterative and proprietary process, with carmakers spending years on the design phase before building out the most promising designs for physical testing. The details and specs of these tests, including aerodynamics data, are typically not made public, slowing down significant advances in performance. MIT engineers sought to fill this data gap by creating a comprehensive dataset of car designs with physically accurate representations of their aerodynamics.
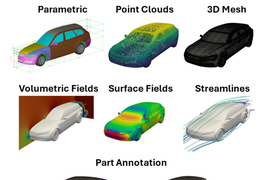
The researchers started with baseline 3D models provided by Audi and BMW and applied a morphing operation to generate distinct car designs. They then ran complex computational fluid dynamics simulations to calculate how air would flow around each design. The resulting dataset contains over 8,000 distinct, physically accurate 3D car forms, encompassing the most common types of passenger cars on the road today.
Applications and Potential Impact
The DrivAerNet++ dataset can be used to train AI models to generate novel car designs with optimized aerodynamics, potentially leading to more fuel-efficient cars and electric vehicles with longer range. The dataset could also be used for the inverse goal, allowing designers to quickly estimate a design’s aerodynamics without physical testing. This could significantly reduce the time and cost associated with car design and development.
“This dataset lays the foundation for the next generation of AI applications in engineering, promoting efficient design processes, cutting R&D costs, and driving advancements toward a more sustainable automotive future,” says Mohamed Elrefaie, a mechanical engineering graduate student at MIT.
The creation of DrivAerNet++ required over 3 million CPU hours using the MIT SuperCloud and generated 39 terabytes of data. The researchers believe that making this dataset publicly available will accelerate innovations in car design and contribute to a more sustainable automotive future.